Jing Wu
Ph.D. Candidate, Monash University
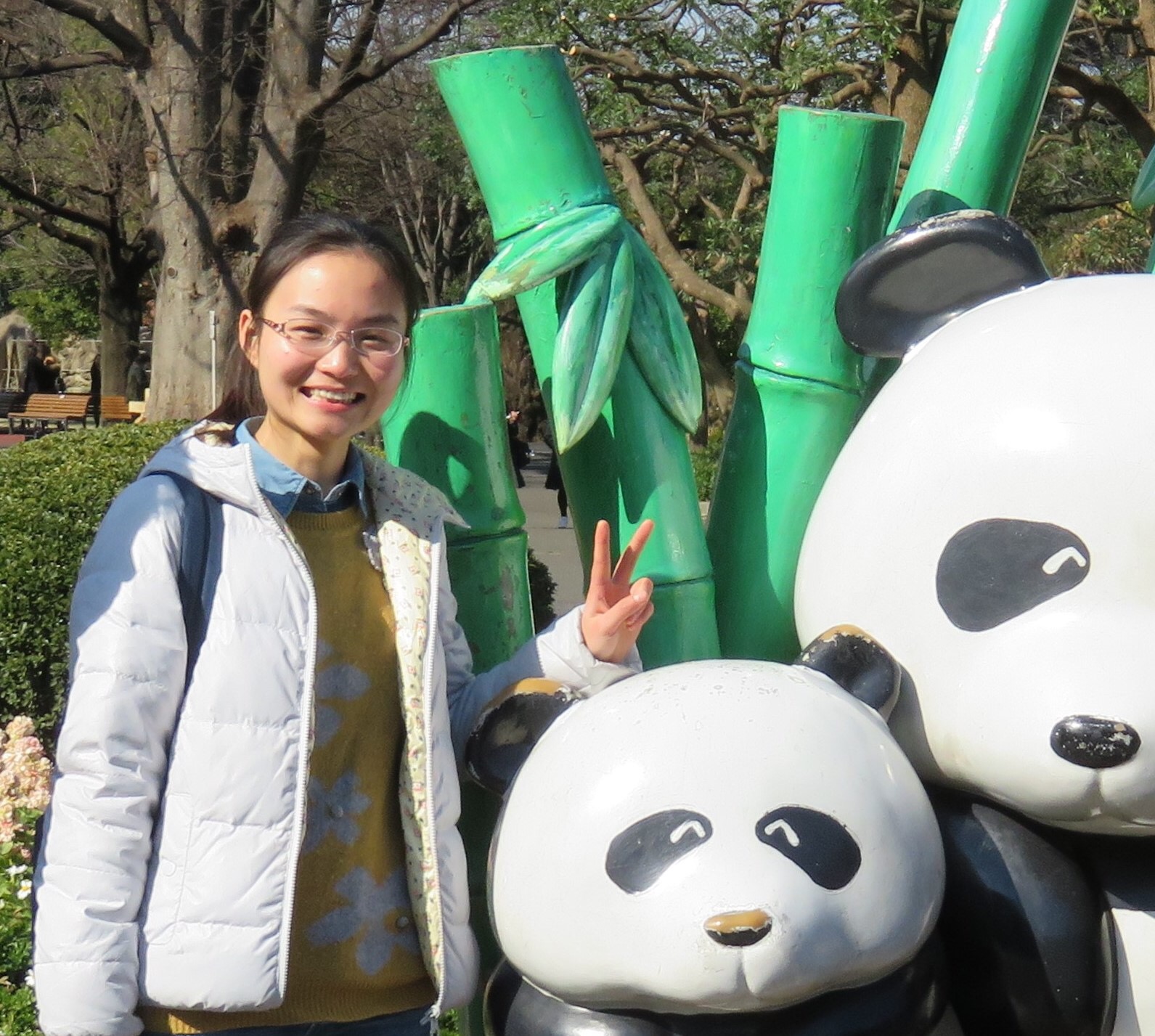
Email: jing.wu1@monash.edu
I am currently a fourth-year Ph.D. student in Engineering at Monash University, Australia, where I have the privilege of being mentored by A/Prof. Mehrtash Harandi and Dr. Munawar Hayat. Before joining Monash University, I was a master’s student at UESTC, where I worked with Prof. Yipeng Liu and Prof. Ce Zhu. I also interned at Megvii under the mentorship of Prof. Shuaicheng Liu.
My work mostly contributes to the fields of Trustworthy ML and Responsible AI. My research mainly focuses on the analysis of the vulnerabilities of deep learning models, and developing algorithms for enhancing safety and reliability by defending against attacks and mitigating inappropriate influence in AI systems. My long-term research objective is to make AI systems safe, reliable, and unbias, as AI increasingly becomes a part of our daily lives, its safety and reliability must be paramount considerations prior to deployment.
selected publications
- MUNBa: Machine Unlearning via Nash BargainingJing Wu, and Mehrtash HarandiarXiv preprint arXiv:2411.15537v2, 2024
Machine Unlearning (MU) aims to selectively erase harmful behaviors from models while retaining the overall utility of the model. As a multi-task learning problem, MU involves balancing objectives related to forgetting specific concepts/data and preserving general performance. A naive integration of these forgetting and preserving objectives can lead to gradient conflicts, impeding MU algorithms from reaching optimal solutions. To address the gradient conflict issue, we reformulate MU as a two-player cooperative game, where the two players, namely, the forgetting player and the preservation player, contribute via their gradient proposals to maximize their overall gain. To this end, inspired by the Nash bargaining theory, we derive a closed-form solution to guide the model toward the Pareto front, effectively avoiding the gradient conflicts. Our formulation of MU guarantees an equilibrium solution, where any deviation from the final state would lead to a reduction in the overall objectives for both players, ensuring optimality in each objective. We evaluate our algorithm’s effectiveness on a diverse set of tasks across image classification and image generation. Extensive experiments with ResNet, vision-language model CLIP, and text-to-image diffusion models demonstrate that our method outperforms state-of-the-art MU algorithms, achieving superior performance on several benchmarks. For example, in the challenging scenario of sample-wise forgetting, our algorithm approaches the gold standard retrain baseline. Our results also highlight improvements in forgetting precision, preservation of generalization, and robustness against adversarial attacks.
@article{wu2024munba, title = {MUNBa: Machine Unlearning via Nash Bargaining}, url = {https://arxiv.org/abs/2411.15537v2}, author = {Wu, Jing and Harandi, Mehrtash}, journal = {arXiv preprint arXiv:2411.15537v2}, year = {2024}, }
- SUN: Training-free Machine Unlearning via SubspaceChengyao Qian, Jing Wu, Trung Le, and 2 more authors2024
Machine Unlearning (MU), a technique to erase undesirable content from AI models, plays an essential role in developing safe and trustworthy AI systems. Despite the success MU achieved, existing MU baselines typically necessitate maintaining the entire dataset for fine-tuning unlearned models. Fine-tuning models and maintaining large datasets are computationally and financially prohibitive. This motivates us to propose a simple yet effective MU approach: \underlineSubspace \underlineUNlearning (SUN) as a new fast and effective MU baseline. The proposed method removes the low-dimensional subspaces of undesirable concepts from the space spanned by the weight vectors. This modification makes the model "blind" to the undesirable content to realize unlearning. Notably, SUN can produce the scrubbed model instantly with only a few samples and without additional training.
@misc{qian2024sun, title = {{SUN}: Training-free Machine Unlearning via Subspace}, author = {Qian, Chengyao and Wu, Jing and Le, Trung and Phung, Dinh and Harandi, Mehrtash}, year = {2024}, url = {https://openreview.net/forum?id=p7mgNvOD9Q}, }
- Erasediff: Erasing data influence in diffusion modelsJing Wu, Trung Le, Munawar Hayat, and 1 more authorIn Proceedings of the IEEE/CVF Conference on Computer Vision and Pattern Recognition, 2025
Diffusion models are highly effective at generating highquality images but pose risks, such as the unintentional generation of NSFW (not safe for work) content. Although various techniques have been proposed to mitigate unwanted influences in diffusion models while preserving overall performance, achieving a balance between these goals remains challenging. In this work, we introduce EraseDiff, an algorithm designed to preserve the utility of the diffusion model on retained data while removing the unwanted information associated with the data to be forgotten. Our approach formulates this task as a constrained optimization problem using the value function, resulting in a natural first-order algorithm for solving the optimization problem. By altering the generative process to deviate away from the groundtruth denoising trajectory, we update parameters for preservation while controlling constraint reduction to ensure effective erasure, striking an optimal trade-off. Extensive experiments and thorough comparisons with state-of-the-art algorithms demonstrate that EraseDiff effectively preserves the model’s utility, efficacy, and efficiency.
@inproceedings{wu2024erasediff, author = {Wu, Jing and Le, Trung and Hayat, Munawar and Harandi, Mehrtash}, booktitle = {Proceedings of the IEEE/CVF Conference on Computer Vision and Pattern Recognition}, title = {Erasediff: Erasing data influence in diffusion models}, year = {2025}, url = {https://arxiv.org/abs/2401.05779}, }
- DaST: Data-Free Substitute Training for Adversarial AttacksMingyi Zhou*, Jing Wu*, Yipeng Liu, and 2 more authorsIn Proceedings of the IEEE/CVF Conference on Computer Vision and Pattern Recognition, 2020
Oral
Machine learning models are vulnerable to adversarial examples. For the black-box setting, current substitute attacks need pre-trained models to generate adversarial examples. However, pre-trained models are hard to obtain in real-world tasks. In this paper, we propose a data-free substitute training method (DaST) to obtain substitute models for adversarial black-box attacks without the requirement of any real data. To achieve this, DaST utilizes specially designed generative adversarial networks (GANs) to train the substitute models. In particular, we design a multi-branch architecture and label-control loss for the generative model to deal with the uneven distribution of synthetic samples. The substitute model is then trained by the synthetic samples generated by the generative model, which are labeled by the attacked model subsequently. The experiments demonstrate the substitute models produced by DaST can achieve competitive performance compared with the baseline models which are trained by the same train set with attacked models. Additionally, to evaluate the practicability of the proposed method on the real-world task, we attack an online machine learning model on the Microsoft Azure platform. The remote model misclassifies 98.35% of the adversarial examples crafted by our method. To the best of our knowledge, we are the first to train a substitute model for adversarial attacks without any real data.
@inproceedings{zhou2020dast, author = {Zhou<sup></sup>, Mingyi and Wu<sup></sup>, Jing and Liu, Yipeng and Liu, Shuaicheng and Zhu, Ce}, booktitle = {Proceedings of the IEEE/CVF Conference on Computer Vision and Pattern Recognition}, title = {DaST: Data-Free Substitute Training for Adversarial Attacks}, year = {2020}, pages = {231-240}, doi = {10.1109/CVPR42600.2020.00031}, }
- Scissorhands: Scrub Data Influence via Connection Sensitivity in NetworksJing Wu, and Mehrtash HarandiIn Computer Vision – ECCV 2024: 18th European Conference, Milan, Italy, September 29–October 4, 2024, Proceedings, Part XLVII, Milan, Italy, 2024
Machine unlearning has become a pivotal task to erase the influence of data from a trained model. It adheres to recent data regulation standards and enhances the privacy and security of machine learning applications. In this work, we present a new machine unlearning approach Scissorhands. Initially, Scissorhands identifies the most pertinent parameters in the given model relative to the forgetting data via connection sensitivity. By reinitializing the most influential top-k percent of these parameters, a trimmed model for erasing the influence of the forgetting data is obtained. Subsequently, Scissorhands fine-tunes the trimmed model with a gradient projection-based approach, seeking parameters that preserve information on the remaining data while discarding information related to the forgetting data. Our experimental results, conducted across image classification and image generation tasks, demonstrate that Scissorhands, showcases competitive performance when compared to existing methods. Source code is available at .
@inproceedings{10.1007/978-3-031-72970-6_21, author = {Wu, Jing and Harandi, Mehrtash}, title = {Scissorhands: Scrub Data Influence via Connection Sensitivity in Networks}, year = {2024}, isbn = {978-3-031-72969-0}, publisher = {Springer-Verlag}, address = {Berlin, Heidelberg}, url = {https://doi.org/10.1007/978-3-031-72970-6_21}, doi = {10.1007/978-3-031-72970-6_21}, booktitle = {Computer Vision – ECCV 2024: 18th European Conference, Milan, Italy, September 29–October 4, 2024, Proceedings, Part XLVII}, pages = {367–384}, numpages = {18}, keywords = {Machine unlearning, Connection sensitivity, Diffusion model}, location = {Milan, Italy}, }
- Concealing Sensitive Samples against Gradient Leakage in Federated LearningJing Wu, Munawar Hayat, Mingyi Zhou, and 1 more authorProceedings of the AAAI Conference on Artificial Intelligence, Mar 2024
Federated Learning (FL) is a distributed learning paradigm that enhances users’ privacy by eliminating the need for clients to share raw, private data with the server. Despite the success, recent studies expose the vulnerability of FL to model inversion attacks, where adversaries reconstruct users’ private data via eavesdropping on the shared gradient information. We hypothesize that a key factor in the success of such attacks is the low entanglement among gradients per data within the batch during stochastic optimization. This creates a vulnerability that an adversary can exploit to reconstruct the sensitive data. Building upon this insight, we present a simple, yet effective defense strategy that obfuscates the gradients of the sensitive data with concealed samples. To achieve this, we propose synthesizing concealed samples to mimic the sensitive data at the gradient level while ensuring their visual dissimilarity from the actual sensitive data. Compared to the previous art, our empirical evaluations suggest that the proposed technique provides the strongest protection while simultaneously maintaining the FL performance. Code is located at https://github.com/JingWu321/DCS-2.
@article{Wu_Hayat_Zhou_Harandi_2024, title = {Concealing Sensitive Samples against Gradient Leakage in Federated Learning}, volume = {38}, url = {https://ojs.aaai.org/index.php/AAAI/article/view/30171}, doi = {10.1609/aaai.v38i19.30171}, number = {19}, journal = {Proceedings of the AAAI Conference on Artificial Intelligence}, author = {Wu, Jing and Hayat, Munawar and Zhou, Mingyi and Harandi, Mehrtash}, year = {2024}, month = mar, pages = {21717-21725}, }
- Analyzing the pregnancy status of giant pandas with hierarchical behavioral informationXianggang Li, Jing Wu, Rong Hou, and 7 more authorsExpert Systems with Applications, Mar 2024
As giant pandas (Ailuropoda melanoleuca) are difficult to conceive and prone to abortion, humans have turned to artificial captive breeding to increase their population. Therefore, it is crucial for their reproduction to analyze their pregnancy status accurately and promptly in artificial captive breeding. To determine whether a giant panda is pregnant, with the current methods, experts must keep a close eye on them and frequently collect their urine or blood, which requires significant resources with a high misdiagnosis rate, and will adversely affect giant pandas’ daily lives. Consequently, it is essential to rapidly advance the development of automated, precise methods that will not disrupt the pandas’ lives to analyze giant pandas’ behaviors and determine whether or not they are pregnant. In this paper, we propose an end-to-end intelligent system for predicting the pregnancy status of giant pandas and their Expected Date of Delivery (EDD). We first introduce expert knowledge to machine learning methods to solve this problem, which can significantly improve the accuracy of prediction. Experimental results show that this system achieves an accuracy of 91.5% for the pregnancy diagnosis and 0.579 days of mean average error for EDD prediction when the observation period is 5 days. Our automated system significantly reduces the need for human intervention, thus minimizing disruptions to the pandas’ daily lives. It has the potential to contribute to the health and genetic diversity of the giant pandas, as well as aid in the panda’s artificial reproduction and population growth.
@article{LI2024121462, title = {Analyzing the pregnancy status of giant pandas with hierarchical behavioral information}, journal = {Expert Systems with Applications}, volume = {237}, pages = {121462}, year = {2024}, issn = {0957-4174}, doi = {https://doi.org/10.1016/j.eswa.2023.121462}, url = {https://www.sciencedirect.com/science/article/pii/S0957417423019644}, author = {Li, Xianggang and Wu, Jing and Hou, Rong and Zhou, Zhangyu and Duan, Chang and Liu, Peng and He, Mengnan and Zhou, Yingjie and Chen, Peng and Zhu, Ce}, keywords = {Giant pandas, Behavior analysis, Neural network, Action recognition, Intelligent video analysis}, }
- Investigating White-Box Attacks for On-Device ModelsMingyi Zhou, Xiang Gao, Jing Wu, and 3 more authorsIn Proceedings of the IEEE/ACM 46th International Conference on Software Engineering, Lisbon, Portugal, Mar 2024
Numerous mobile apps have leveraged deep learning capabilities. However, on-device models are vulnerable to attacks as they can be easily extracted from their corresponding mobile apps. Although the structure and parameters information of these models can be accessed, existing on-device attacking approaches only generate black-box attacks (i.e., indirect white-box attacks), which are less effective and efficient than white-box strategies. This is because mobile deep learning (DL) frameworks like TensorFlow Lite (TFLite) do not support gradient computing (referred to as non-debuggable models), which is necessary for white-box attacking algorithms. Thus, we argue that existing findings may underestimate the harm-fulness of on-device attacks. To validate this, we systematically analyze the difficulties of transforming the on-device model to its debuggable version and propose a Reverse Engineering framework for On-device Models (REOM), which automatically reverses the compiled on-device TFLite model to its debuggable version, enabling attackers to launch white-box attacks. Our empirical results show that our approach is effective in achieving automated transformation (i.e., 92.6%) among 244 TFLite models. Compared with previous attacks using surrogate models, REOM enables attackers to achieve higher attack success rates (10.23%→89.03%) with a hundred times smaller attack perturbations (1.0→0.01). Our findings emphasize the need for developers to carefully consider their model deployment strategies, and use white-box methods to evaluate the vulnerability of on-device models. Our artifacts 1 are available.
@inproceedings{10.1145/3597503.3639144, author = {Zhou, Mingyi and Gao, Xiang and Wu, Jing and Liu, Kui and Sun, Hailong and Li, Li}, title = {Investigating White-Box Attacks for On-Device Models}, year = {2024}, isbn = {9798400702174}, publisher = {Association for Computing Machinery}, address = {New York, NY, USA}, url = {https://doi.org/10.1145/3597503.3639144}, doi = {10.1145/3597503.3639144}, booktitle = {Proceedings of the IEEE/ACM 46th International Conference on Software Engineering}, articleno = {152}, numpages = {12}, location = {Lisbon, Portugal}, series = {ICSE '24}, }
- ModelObfuscator: Obfuscating Model Information to Protect Deployed ML-Based SystemsMingyi Zhou, Xiang Gao, Jing Wu, and 4 more authorsIn Proceedings of the 32nd ACM SIGSOFT International Symposium on Software Testing and Analysis, Seattle, WA, USA, Mar 2023
More and more edge devices and mobile apps are leveraging deep learning (DL) capabilities. Deploying such models on devices – referred to as on-device models – rather than as remote cloud-hosted services, has gained popularity because it avoids transmitting user’s data off of the device and achieves high response time. However, on-device models can be easily attacked, as they can be accessed by unpacking corresponding apps and the model is fully exposed to attackers. Recent studies show that attackers can easily generate white-box-like attacks for an on-device model or even inverse its training data. To protect on-device models from white-box attacks, we propose a novel technique called model obfuscation. Specifically, model obfuscation hides and obfuscates the key information – structure, parameters and attributes – of models by renaming, parameter encapsulation, neural structure obfuscation, shortcut injection, and extra layer injection. We have developed a prototype tool ModelObfuscator to automatically obfuscate on-device TFLite models. Our experiments show that this proposed approach can dramatically improve model security by significantly increasing the difficulty of parsing models’ inner information, without increasing the latency of DL models. Our proposed on-device model obfuscation has the potential to be a fundamental technique for on-device model deployment. Our prototype tool is publicly available at https://github.com/zhoumingyi/ModelObfuscator.
@inproceedings{10.1145/3597926.3598113, author = {Zhou, Mingyi and Gao, Xiang and Wu, Jing and Grundy, John and Chen, Xiao and Chen, Chunyang and Li, Li}, title = {ModelObfuscator: Obfuscating Model Information to Protect Deployed ML-Based Systems}, year = {2023}, isbn = {9798400702211}, publisher = {Association for Computing Machinery}, address = {New York, NY, USA}, url = {https://doi.org/10.1145/3597926.3598113}, doi = {10.1145/3597926.3598113}, booktitle = {Proceedings of the 32nd ACM SIGSOFT International Symposium on Software Testing and Analysis}, pages = {1005–1017}, numpages = {13}, keywords = {AI safety, SE for AI, model deployment, model obfuscation}, location = {Seattle, WA, USA}, series = {ISSTA 2023}, }
- DaST: Data-Free Substitute Training for Adversarial AttacksMingyi Zhou*, Jing Wu*, Yipeng Liu, and 2 more authorsIn Proceedings of the IEEE/CVF Conference on Computer Vision and Pattern Recognition, Mar 2020
Oral
Machine learning models are vulnerable to adversarial examples. For the black-box setting, current substitute attacks need pre-trained models to generate adversarial examples. However, pre-trained models are hard to obtain in real-world tasks. In this paper, we propose a data-free substitute training method (DaST) to obtain substitute models for adversarial black-box attacks without the requirement of any real data. To achieve this, DaST utilizes specially designed generative adversarial networks (GANs) to train the substitute models. In particular, we design a multi-branch architecture and label-control loss for the generative model to deal with the uneven distribution of synthetic samples. The substitute model is then trained by the synthetic samples generated by the generative model, which are labeled by the attacked model subsequently. The experiments demonstrate the substitute models produced by DaST can achieve competitive performance compared with the baseline models which are trained by the same train set with attacked models. Additionally, to evaluate the practicability of the proposed method on the real-world task, we attack an online machine learning model on the Microsoft Azure platform. The remote model misclassifies 98.35% of the adversarial examples crafted by our method. To the best of our knowledge, we are the first to train a substitute model for adversarial attacks without any real data.
@inproceedings{zhou2020dasu, author = {Zhou<sup></sup>, Mingyi and Wu<sup></sup>, Jing and Liu, Yipeng and Liu, Shuaicheng and Zhu, Ce}, booktitle = {Proceedings of the IEEE/CVF Conference on Computer Vision and Pattern Recognition}, title = {DaST: Data-Free Substitute Training for Adversarial Attacks}, year = {2020}, pages = {231-240}, doi = {10.1109/CVPR42600.2020.00031}, }